How 'observability' can keep your data lake clean
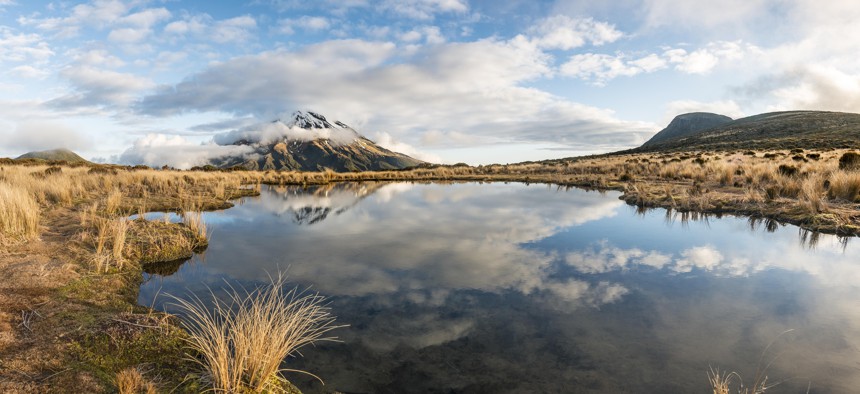
Gettyimages.com/ Moritz Wolf
The use of data lakes is growing in the federal market, and they have great potential for better analysis and data-driven decision-making -- as long as they are kept clean and pollution-free.
When it comes to data security in the federal government, most people think about technology such as data-at-rest or data-in-motion encryption in order to prevent bad actors from introducing viruses or otherwise taking control of data.
But with the growing acceptance of so-called “data lakes” in government technology, data encryption has become only one part of the data security arsenal.
In fact, there’s a new category of federal data security technology: “data observability.” This technology has one main and increasingly vital function, which is to keep data lakes from being polluted. Agencies and contractors alike would do well to understand the problems arising from polluted data lakes and how data observability can help.
The concept of data lakes clearly is catching on in the federal government, with large-scale plans announced as far back as 2020 by both the Census Bureau and the Defense Department. Other agencies and sub-agencies also are taking a close look at the technology for their own purposes.
The Census Bureau’s enterprise data lake, estimated to cost $22.3 million, is planned to increase their capacity for administrative, economic and demographic data. IT leaders at the Census Bureau believe the technology will enable modernization of data storage and improved data analytics capabilities.
In May 2021, the DOD issued the Five Decrees that in part states how a “data-centric organization is critical to improving performance and creating decision advantage at all echelons from the battlespace to the board room.” The expectation is that data is and will continue to be a competitive advantage for our nation.
The Joint All-Domain Command and Control concept is a novel approach that fundamentally relies on sharing data-in-motion between various services and components.
One word is key in all of these cases: Analytics. The advantages of a data lake are almost always tied to increased use of artificial intelligence for analysis. After all, it could take multiple lifetimes for individuals to analyze the volumes of data in a data lake on their own. The data lake is of little value without the models for AI interpretation.
Data lakes bring up a very pastoral image. But what happens when that data lake becomes polluted -- whether by malicious or unintentional errors introduced into data files? AI models built on polluted data lakes cannot be trusted to produce meaningful analysis. That can result in lost productivity, increased costs or worse consequences.
There are several ways in which a data lake can become polluted. These underscore the need for data observability from the very earliest stages of creating a data lake:
Deficient data volumes
It’s reasonably easy to determine how healthy your data flow is by monitoring file counts through processes like batch Extract-Transform-Load. If you can determine whether a specific number of files is missing over a set period of time, you can similarly determine whether an entire volume of expected data has been received.
Fewer files means an upstream data supply chain problem. More files than expected can mean duplicate data.
Possible data corruption
It is essential to observe structured data schema for aberrations. Data where it isn’t expected, even something as innocent as formatting errors leading to extra blank columns, can cause problems down the line.
You can avoid downstream analytical production problems caused by subtle flaws in analytics by using data quality monitoring and data observability as early in the process as possible.
Incomplete data
Any given file can contain potentially billions of data points. That can become disastrous for machine learning models when densely-packed data includes empty or null values. If many data points have empty fields, training data models can be untrustworthy and analysis becomes faulty.
Data observability can measure how many null values are being recorded as a percentage of total data. When measured against a performance monitoring baseline, you establish a way to warn in real time if a potential problem is starting.
Duplicative data
It is expensive to store duplicate data, and it can also create biased outcomes for analytics and machine learning. Knowing if your data is duplicative can cut operational costs dramatically.
Late data
Late data is a real problem for organizations that have already conducted transformations, aggregations and analysis. When data arrives late, it also means that AI models built with the incomplete data may be unreliable.
The concept of data lakes has not taken hold in the federal government to the same extent it has in the private sector, but that is actually an operational advantage for agencies and the vendor community that serves them.
Little good happens when applying AI to data lakes to find out after the fact that pollution is there. By employing data observability at the outset of a major data lake initiative, you can set measurable parameters to determine up front whether the behavior of data filling your data lake will cause potential problems.
We’re well positioned now to be proactive about the quality and data security of our data lakes. All that’s left is to start applying methods and practices now -- before the AI modeling begins.
Dave Hirko is founder and principal of Zectonal. He can be reached at dave@zectonal.com